
A new machine-learning system co-created by an Indian-origin researcher at Massachusetts Institute of Technology (MIT) can determine outcomes in hospitals by taking into account medical data, including electronic health records, to help doctors make better medical decisions.
Created jointly by Harini Suresh and her team at MIT’s Computer Science and Artificial Intelligence Laboratory (CSAIL), the system called “ICU Intervene” takes large amounts of intensive-care-unit (ICU) data, from vitals and labs to notes and demographics, to determine what kinds of treatments are needed for different symptoms.

It uses “deep learning” to make real-time predictions, learning from past ICU cases to make suggestions for critical care, while also explaining the reasoning behind these decisions.

“The system could potentially be an aid for doctors in the ICU, which is a high-stress, high-demand environment,” said Suresh, the lead author on the paper about ICU Intervene. “The goal is to leverage data from medical records to improve health care and predict actionable interventions,” she added.

Another team of researchers developed an approach called “EHR Model Transfer” that can facilitate the application of predictive models on an electronic health record (EHR) system, despite being trained on data from a different EHR system. Specifically, using this approach the team showed that predictive models for mortality and prolonged length of stay can be trained on one EHR system and used to make predictions in another.
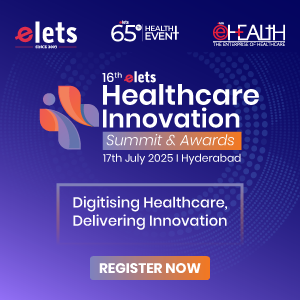
While Suresh and her team presented ICU Intervene at the Machine Learning for Healthcare Conference in Boston earlier this month, EHR Model Transfer was presented recently at the ACM’s Special Interest Group on Knowledge Discovery and Data Mining in Halifax, Canada.
Both the models will be of immense help to doctors, who are often deluged by signals from charts, test results, and other metrics to keep track of. They also find is extremely difficult to integrate and monitor huge amount of data for multiple patients while making real-time treatment decisions, especially when data is documented inconsistently across hospitals.
Be a part of Elets Collaborative Initiatives. Join Us for Upcoming Events and explore business opportunities. Like us on Facebook , connect with us on LinkedIn and follow us on Twitter , Instagram.
"Exciting news! Elets technomedia is now on WhatsApp Channels Subscribe today by clicking the link and stay updated with the latest insights!" Click here!