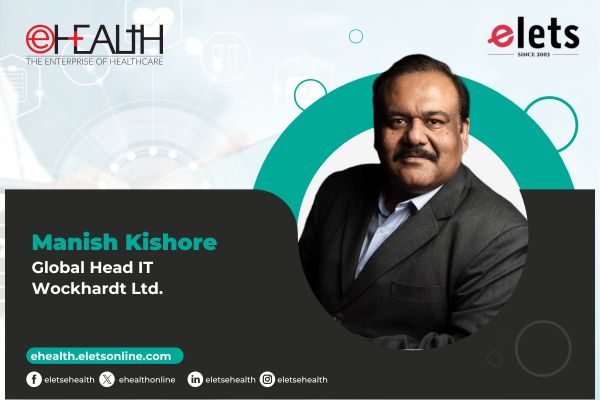
India’s healthcare sector is at a critical juncture. Despite recent advancements, a significant portion of the population (approximately 63%, according to WHO data) lacks access to essential medicines. However, a transformative solution is emerging – the convergence of Artificial Intelligence (AI) and digital technologies. This offers the potential to bridge the healthcare gap, addressing the unique challenges posed by India’s large and diverse population, and to reshape healthcare accessibility, efficiency, and patient outcomes across urban and rural areas. Initiatives such as the Ayushman Bharat Digital Mission (ABDM) are pivotal in this digital evolution, paving the way for data-driven healthcare delivery.
Impact and Potential Due to Increasing Use of AI, and Digital Technologies
- Improved Accessibility: Digital health solutions are expanding access to healthcare services, especially in remote areas.
- Enhanced Efficiency: Digitalization is streamlining administrative processes and improving the efficiency of healthcare delivery.
- Data-Driven Decision Making: AI-powered analytics and machine learning are enabling enhanced clinical decision-making and the development of personalized care plans.
- Increased Affordability: Digital health can help reduce healthcare costs by improving efficiency and reducing the need for physical visits.
- Global Recognition: India’s digital health efforts are gaining international recognition, with the country being seen as a potential leader in this field.
The Transformative Role of AI in Indian Healthcare
AI is rapidly assuming a central role in reshaping healthcare delivery in India, addressing key challenges that include

- Limited access to specialists
- High disease burden
- Inefficiencies within healthcare systems
Major Areas of Significant AI Impact
Diagnostics and Imaging
AI-driven diagnostic systems, employing sophisticated deep learning architectures such as Convolutional Neural Networks (CNNs), Transformers, and Generative Adversarial Networks (GANs), are significantly augmenting the precision and efficiency of medical image analysis.
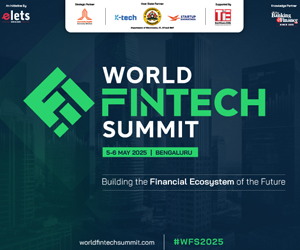
These methodologies facilitate accelerated and refined diagnoses, particularly critical in regions with a high disease burden, including tuberculosis and oncological pathologies. Specifically, deep learning models, leveraging pre-trained weights and transfer learning techniques, are demonstrating exceptional performance in retinal image analysis for the early detection of diabetic retinopathy. These systems achieve diagnostic accuracies that are statistically non inferior, and in some instances, superior, to those of human ophthalmologists, quantified through metrics such as sensitivity, specificity, and area under the receiver operating characteristic curve (AUC-ROC).

Furthermore, the integration of attention mechanisms and explainable AI (XAI) techniques within these systems allows for the visualization and interpretation of the model’s decision-making process, enhancing clinical trust and facilitating the identification of subtle pathological features.
Diagnostic algorithms, leveraging supervised learning paradigms and transfer learning methodologies applied to extensive, heterogeneous medical image datasets, facilitate the identification of pathological conditions such as tuberculosis, oncological lesions, and diabetic retinopathy from modalities including radiographic (X-ray), computed tomography (CT), and magnetic resonance imaging (MRI). This deployment is particularly impactful in geographically isolated rural communities characterized by limited access to specialized radiological expertise.
The provision of AI-generated preliminary diagnostic interpretations enables expedited patient triage and informed clinical decision-making, thereby addressing critical healthcare accessibility disparities prevalent in India, where specialist services are predominantly concentrated in urban centres. However, the robustness and generalizability of these AI systems are contingent upon the quality and representativeness of the training data.
Algorithmic biases, arising from underrepresentation of specific demographic or clinical subpopulations, can lead to diagnostic inaccuracies. To mitigate these risks, the implementation of stringent data curation protocols, including rigorous image pre-processing, expert-driven annotation, and cross-validation techniques, is imperative.
Furthermore, the incorporation of fairness-aware machine learning strategies is crucial to ensure equitable performance across diverse patient populations.
Personalized Medicine
Advanced analytical methodologies, leveraging machine learning algorithms and deep neural networks, are being deployed to interrogate multi-modal patient datasets for personalized medicine applications. These datasets encompass:
- Genomic Data: Analyzed via sophisticated bioinformatics pipelines, including variant calling, gene expression profiling, and pathway analysis.
- Clinical Phenotypic Data: Extracted from Electronic Health Records (EHRs) using Natural Language Processing (NLP) for information retrieval, entity recognition, and relationship extraction.
- Environmental and Behavioral Data: Acquired from wearable sensor arrays and validated patient-reported outcome measures, providing insights into lifestyle and physiological parameters.
The integration and analysis of these heterogeneous data streams facilitate the development of individualized therapeutic strategies, optimizing treatment efficacy based on unique patient profiles.
This personalized medicine paradigm, driven by AI’s capacity for complex pattern recognition and predictive modeling of treatment response, aims to maximize therapeutic outcomes and minimize adverse drug reactions through precise drug selection and dosage optimization. However, the ethical imperatives of data privacy and security are paramount.
Robust governance frameworks, incorporating stringent data anonymization protocols, informed consent mechanisms, and secure data storage and transmission architectures, are essential to ensure patient trust and regulatory compliance.
Drug Discovery and Development
AI is substantially accelerating the drug discovery and development pipeline through several key applications:
- Computational Molecular Modeling and Interaction Prediction: Graph Neural Networks (GNNs) and other deep learning architectures are employed to analyze molecular structures, predicting their physicochemical properties and potential interactions with biological targets.
These models enable the in silico prediction of ligand-protein binding affinities and binding modes, facilitating the identification of promising drug candidates.
- Predictive Pharmacology and Toxicology: Machine learning models, trained on extensive datasets comprising compound structures and pharmacological/toxicological profiles, are utilized to predict drug efficacy and potential toxicity. This application aims to minimize attrition rates in clinical trials by prioritizing compounds with favorable safety and efficacy profiles.
- Target Discovery and Pathway Analysis: Knowledge graph embeddings and network biology approaches are applied to analyze large-scale biological datasets, including transcriptomic, proteomic, and protein-protein interaction networks, to identify novel molecular targets for therapeutic intervention. These methodologies can reveal previously unrecognized disease-associated proteins or pathways, enabling the development of targeted therapies.
- Drug Repositioning and Polypharmacology: AI algorithms are leveraged to analyze drug-target interaction databases and clinical trial data, identifying potential new indications for existing drugs. This approach accelerates the availability of repurposed drugs for novel therapeutic applications.
The integration of AI-driven methodologies into the drug discovery process has the potential to significantly reduce the time and cost associated with bringing new treatments to market, addressing urgent healthcare needs with greater efficiency. In silico screening and computational modeling can drastically reduce the number of compounds requiring physical synthesis and in vitro/in vivo testing. However, ensuring equitable access to AI-developed pharmaceuticals is paramount. Policy frameworks must be established to prevent the exacerbation of existing healthcare disparities and ensure that the benefits of AI-driven drug discovery are distributed fairly.
Remote Patient Monitoring
AI-integrated wearable sensor platforms and mobile health applications are enabling continuous physiological monitoring and remote patient surveillance, particularly advantageous for the longitudinal management of chronic diseases.
- Real-time Physiological Data Analytics: Advanced time-series analysis and machine learning models, including recurrent neural networks (RNNs) and long short term memory (LSTM) networks, are employed to process data streams from wearable sensors (e.g., smartwatches, fitness trackers, continuous glucose monitors).
These algorithms detect temporal patterns and anomalies in vital signs, such as heart rate variability (HRV), blood pressure, glucose fluctuations, and sleep architecture. Predictive analytics, utilizing these processed data, facilitate the early detection of potential cardiac events or impending diabetic crises. - Proactive Clinical Intervention and Risk Mitigation: AI-driven alert systems provide real-time notifications to patients and healthcare providers upon the detection of critical deviations in monitored physiological parameters, enabling timely clinical intervention and mitigating the risk of acute exacerbations.
Predictive modelling, leveraging machine-learning algorithms, identifies patients at elevated risk for hospital readmission based on longitudinal physiological data and clinical covariates, facilitating targeted post-discharge care and support.
The inherent variability and potential noise in wearable sensor data necessitate the implementation of robust data pre-processing pipelines, including signal filtering, artifact removal, and imputation techniques, to ensure the reliability and validity of AI-driven analytics.
Chatbots and Virtual Assistants
Conversational AI platforms, powered by Natural Language Processing (NLP) and machine learning models, are enabling real-time patient engagement and information dissemination. These platforms provide on-demand access to medical information, including medication instructions and pre-appointment guidelines, facilitate automated appointment scheduling, and address basic health-related inquiries, such as symptom triage and navigational assistance.
These tools enhance patient-provider communication, augment patient engagement and satisfaction, and alleviate the administrative workload of healthcare professionals, enabling them to focus on complex clinical tasks.
Furthermore, AI-driven chatbots are being deployed to provide continuous, 24/7 support for patients with mental health conditions, offering a secure and accessible avenue for information and resource access. The development of culturally competent conversational AI agents is crucial, necessitating the integration of multilingual capabilities and sensitivity to socio-cultural nuances to ensure effective communication across diverse patient populations.
Key Drivers and Initiatives
- ABDM and Data-Driven Decision-Making: The Ayushman Bharat Digital Mission (ABDM) is a pivotal initiative aimed at creating a unified digital health ecosystem in India, fostering interoperability and data-led healthcare delivery. Major aspects of the ABDM include ABHA (Ayushman Bharat Health Account), Health Facility Registry (HFR) and Healthcare Professionals Registry (HPR) and Unified Health Interface (UHI).
- Digital Public Infrastructure (DPI): India’s robust DPI is a crucial enabler and foundational digital systems that are accessible, secure, and interoperable, supporting essential public services. In India, DPI has been instrumental in transforming the digital economy, much like traditional infrastructure for growth.
- Telemedicine and eSanjeevani: Telemedicine platforms are bridging geographical barriers, particularly in rural and underserved areas. eSanjeevani is a prime example of a telemedicine service that is providing healthcare to millions of people.
- Tech Enablers like AI and Data Analytics: Artificial intelligence and advanced data analytics serve as critical enablers for the digital healthcare transformation in India, underpinning the functionality and effectiveness of initiatives like ABDM and telemedicine platforms. AI-powered tools are integral to enhanced diagnostics, personalized treatment plans, operational efficiency, and predictive analytics, while data analytics platforms are crucial for population health management, healthcare resource optimization, and clinical decision support.
The integration of these technologies into the digital health ecosystem is essential for realizing the full potential of initiatives such as ABDM and telemedicine, contributing to more efficient, accessible, and equitable healthcare delivery across India.
- Government Initiatives: The Indian government is actively promoting digital health through policies and investments. Initiatives like the National Health Policy and the National Digital Health Mission are driving the adoption of digital technologies in healthcare.
Future Trends in Digital Healthcare
Based on reports from the World Economic Forum (WEF) and the World Health Organization (WHO), several major trends are poised to shape the future of digital healthcare in India:
- Accelerated AI Integration: AI is expected to accelerate significant advancements across all areas of healthcare delivery, with investments in AI within the healthcare industry projected to reach $11.78 billion by 2025.
This technology is anticipated to enhance diagnostic capabilities, improve operational efficiency (e.g., automating administrative tasks), and contribute to improved patient outcomes.
- Expansion of Telemedicine Services: The utilization of telemedicine services is expected to continue its significant expansion, maintaining its crucial role in broadening healthcare access, particularly in underserved regions.
Initiatives such as India’s eSanjeevani Telemedicine Service have demonstrated telemedicine’s potential to facilitate millions of consultations and extend healthcare reach. Telemedicine is particularly important in India, where geographical barriers can significantly hinder access to care.
- Development of Digital Health Ecosystems: The Ayushman Bharat Digital Mission (ABDM) is leading the development of a more interconnected digital health infrastructure, aiming to enhance interoperability among healthcare providers and provide a seamless healthcare experience for patients.
This includes the integration of various digital health tools and platforms to create a cohesive and user-friendly system.
- Emphasis on Data-Driven Healthcare: The increasing application of digital tools for data analytics is transforming healthcare systems by optimizing costs and improving treatment effectiveness.
AI-based analytics is likely to become a key driver in enabling personalized medicine strategies and predictive diagnostics, leading to more targeted and effective interventions.
- Global Collaboration and Standardization: The World Health Organization (WHO) is advocating for increased global collaboration in the development of digital health policies, with a focus on interoperability, security, and data-driven policy applications to advance global healthcare.
This is crucial for ensuring that digital health solutions are safe, effective, and can be readily shared and adapted across different countries.
Challenges and Strategic Mitigation for Equitable Digital Healthcare Deployment in India
Despite the transformative potential of digital health technologies, India faces several critical challenges that must be addressed to ensure equitable and effective implementation:
- Data Confidentiality and Cybersecurity:The safeguarding of sensitive Protected Health Information (PHI) is of paramount importance. This necessitates the implementation of robust data governance frameworks, incorporating end-to-end encryption, access control mechanisms, and intrusion detection systems, to protect patient trust and ensure adherence to regulatory mandates, such as the Digital Personal Data Protection (DPDP) Act.
- Digital Inclusion and Infrastructure Development: Bridging the digital divide through the expansion of broadband internet connectivity, particularly in underserved and rural areas, is essential.
Concurrently, fostering digital literacy among both patients and healthcare providers through targeted educational programs and training initiatives is critical for equitable access to digital healthcare services.
- Interoperability and Data Exchange Standards: The development and implementation of standardized data exchange protocols, such as HL7 FHIR, are crucial to enable seamless integration and interoperability across heterogeneous healthcare information systems and platforms.
This facilitates secure and efficient sharing of patient data, enhancing care coordination and clinical decision support.
- Ethical Governance of AI in Healthcare: Addressing the ethical implications of AI deployment in healthcare is imperative. This encompasses the mitigation of algorithmic biases through fairness-aware machine learning techniques, the implementation of robust informed consent protocols for AI-driven interventions, and the establishment of transparent AI decision-making frameworks to ensure accountability and build public trust. Additionally, continuous auditing of deployed AI systems is needed.
The Road Ahead
India is positioned at the vanguard of a transformative digital healthcare paradigm, wherein the synergistic integration of artificial intelligence and data-driven methodologies is poised to fundamentally reshape healthcare delivery.
Strategic initiatives, such as the ABDM are establishing a robust digital infrastructure to facilitate the development of an accessible, efficient, and equitable healthcare ecosystem.
While significant advancements have been achieved in areas including AI-enhanced diagnostics, precision medicine, and computational drug discovery, the proactive mitigation of critical challenges—encompassing data confidentiality and cybersecurity, digital inclusion and infrastructure development, interoperability and data exchange standardization, and the ethical governance of AI— remains paramount.
By implementing comprehensive mitigation strategies, fostering interdisciplinary collaboration among stakeholders and adhering to rigorous regulatory frameworks, India can effectively leverage the full potential of digital health technologies to substantially enhance the health outcomes of its diverse populace and serve as a pioneering model for global healthcare transformation.
Views expressed by: Manish Kishore, Global Head IT, Wockhardt Ltd.
Be a part of Elets Collaborative Initiatives. Join Us for Upcoming Events and explore business opportunities. Like us on Facebook , connect with us on LinkedIn and follow us on Twitter , Instagram.
"Exciting news! Elets technomedia is now on WhatsApp Channels Subscribe today by clicking the link and stay updated with the latest insights!" Click here!